Invited speakers
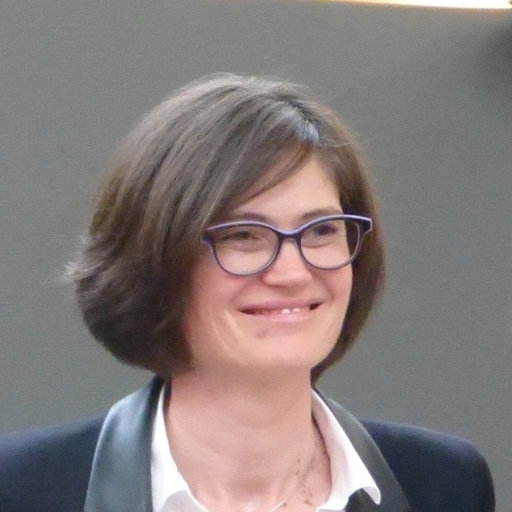
Cécile Bothorel : Alignment and stability of embeddings
Representation learning methods learn latent embeddings of objects where the information is preserved by the distances. Since distances are invariant to certain linear transformations, one can obtain different embeddings while preserving the same information. In dynamical systems, a temporal difference in embeddings can be explained by the misalignment of embeddings due to such arbitrary transformations, but also by the intrinsic instability of the system. We explore the alignment of the embeddings and its parts, propose new metrics to measure both alignment and stability, and show how realignment improves the performance of inference tasks in dynamic networks.
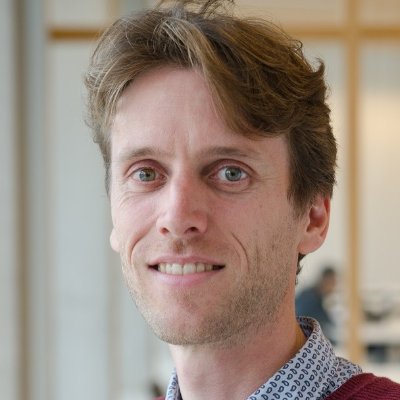
Tijl De Bie : Trustworthy network embedding: fairness, robustness, explainability, and evaluation - and an application to the job market
Network embedding methods have become standard tools for learning useful representations of nodes in networks. Until recently, however, their usefulness has been evaluated mainly in terms of the accuracy of downstream tasks that make use of them. In this talk, I will discuss several other important criteria to evaluate the trustworthiness of network embeddings, including how to limit bias in such embeddings, how to ensure they are robust, how to ensure some degree of transparency, and how to evaluate them. I will discuss these criteria based on concrete algorithmic examples, and illustrate them on an application to the job market: the development of a trustworthy job-to-applicant recommender system.